Dec 20, 2023Researchers study AI for thinning, pruning automation
Researchers are examining ways AI can help improve orchard thinning and pruning.
Oregon State University (OSU) and Washington State University scientists with AI, mechanical engineering and computer science expertise are also considering how the tech they’re studying can apply to other crops, including berries.
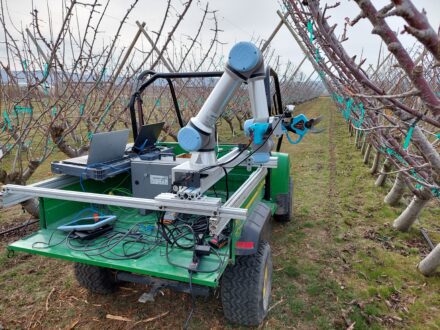
During the FIRA USA robotics in agriculture conference in Salinas, California, Joe Davidson, assistant professor of robotics and mechanical engineering at OSU’s School of Mechanical, Industrial and Manufacturing Engineering (MIME), gave an overview of robotic pruning research through the AgAID Institute (USDA-NIFA Institute for Agricultural AI for Transforming Workforce and Decision Support).
The new organization is a National Science Foundation institute funded by the USDA-National Institute of Food and Agriculture. Institute members also include University of California-Merced, University of Virginia, Heritage University, Wenatchee Valley College, Kansas State University, IBM Research and innov8.ag.
Employing advanced AI techniques to build human robot collaborative solutions that strengthen and amplify orchard workers, the researchers want to improve fruit quality and yield as well as increase labor productivity. Davidson works in OSU’s Intelligent Machines and Materials Lab and Collaborative Robotics and Intelligent Systems Institute in Corvallis, Oregon.
In addition to AI research, the Institute studies water, farm and labor intelligence. Because fresh market fruit production is labor intensive, it requires complex decision making and generally receives less attention from private companies. Researchers chose to focus on robotic thinning and pruning because of the lack of attention to those practices.
Davidson and his colleagues have collected extensive data from apple and sweet cherry orchards. Because those environments favor automation, researchers concentrate on high-density, planar orchard systems.
The researchers want to use digital data to train perception models for computer vision and robot controllers.
AI pruning vision
In a field trial in Prosser, Washington, last winter, a general purpose manipulator mounted on an operator driven utility vehicle included an eye-in-hand configuration. The color depth camera mounted at the end of the robot was used to identify pruning points. Electric bypass shears autonomously cut limbs. The machinery taught researchers much.
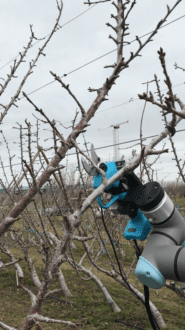
To drive a branch into the cutting implement, a digital network receives an RGB image, known as a “truecolor image” that defines red, green and blue color components. That visual feedback from the camera instructs the machinery to move left, right, up or down, to drive the branch to the tool’s fulcrum point to maximize the likelihood of a successful cut.
The algorithm trained by digital data performs as well as those trained with human annotated data. Scientists can create AI deep learning models more robust than those trained only using hand-labeled data, Davidson said.
When more variables are present in the environment, including different orchard conditions, tree architecture and weather, software trained in simulation using generative AI is stronger across the varying conditions, he said. A different time of the day with similar environmental lighting conditions produces high similarities. However, cloudy conditions with different light can present challenges, as would different orchards with other tree types.
Vision capabilities, however, aren’t enough. Vision needs to be combined with touch if a grower is performing precision cuts on small thin branches, and risks touching other branches. Using a hybrid control that also provides visual feedback on what an operator actually sees in the environment plus force feedback — the sense of touch needed to drive the branch to the correct location of the cutting tool — are necessary, Davidson said.
Creating digital trees
The effort emphasizes the practical vs. large integrated trials at scale.
“We have made a deliberate decision to go to the field early and to work on system integration and do preliminary field trials,” Davidson said. “We want to identify where our ideas were viable and where we should go back to the drawing board.”
To label training data for deep learning algorithms, researchers used open-source tools to mark images of trees or orchards in dormancy. The process, however, is time intensive and even if a knowledgeable individual performs the annotation, errors can still occur in determining what the image is showing the viewer and if the branch photographed is a vigorous limb or a spur. If a human looks at an image of a tree in dormancy, it’s difficult to determine if the branch belongs to a certain tree or if it is the one in the foreground or background, Davidson said.
“There are tools out there where you can create digital trees, using realistic growth models with light distribution, carbon transport and all the things horticulturists care about and study,” Davidson said. “You can actually create digital environments where you can train robot controllers.”
Reinforcement learning can train a robot to use visual feedback from a camera for precision pruning. In orchard images, scientists want to segment or isolate pixels from a tree in front of the robot, the one a grower wants to prune, from trees in the background. Trained networks perform the task using synthetic data and a technique known as optical flow. Digitally, patterns, colors and textures of objects in the scene can be continuously changed to make the algorithms more precise.
Next steps in the research involve studies with professional pruners this winter. Davidson has been working on digital training tools that growers can use with their seasonal workforce to help them get on board when it comes to pruning rules and requirements.
Davidson and his colleagues are expanding the technology to different crops, including pruning blueberries, which are a significant crop in Oregon and Washington.
They are also applying methods and techniques developed for robotic pruning to other orchard activities, including automatic tree trunk cross sectional area measurement and measuring canopy volume, color changes and vigor for a precision orchard fertilization project.
In addition to the tech, human studies are also conducted, including interviews and observational studies of orchard managers. Co-leading the labor intelligence effort, Davidson spends most of his research time on labor intelligence.
— By Doug Ohlemeier, assistant editor